The future of machine learning is increasingly focused on providing personalized experiences. As companies across the globe scramble to offer individualized services to users, large language models (LLMs) have emerged as a powerful tool for achieving this goal. The use of LLMs, however, brings forth the concern of computational cost and efficiency, especially when dealing with huge data sets.
At Particlesy, our advanced AI platform leverages a ground-breaking approach known as Retrieval-Augmented Generation (RAG) to address these issues. RAG combines the benefits of retrieval-based models and sequence-to-sequence generative models, offering an economically viable solution for personalization.
In this article, we’ll delve into:
- The Need for Personalized LLM Experiences
- The Shortcomings of Traditional LLM Approaches
- What is Retrieval-Augmented Generation (RAG)?
- How RAG Makes Personalization Cost-Effective
- The Future of Personalization with RAG
The Need for Personalized LLM Experiences
Personalization in machine learning is not a novel concept. The general aim is to provide user-specific services or responses, enhancing user engagement and satisfaction. Research by McKinsey & Company has shown that personalization can deliver five to eight times the ROI on marketing spend, and can lift sales by 10% or more.
In the realm of LLMs, personalization could mean generating responses that take into account the user’s past interactions, preferences, or other personalized data, thereby offering an almost human-like interaction experience.
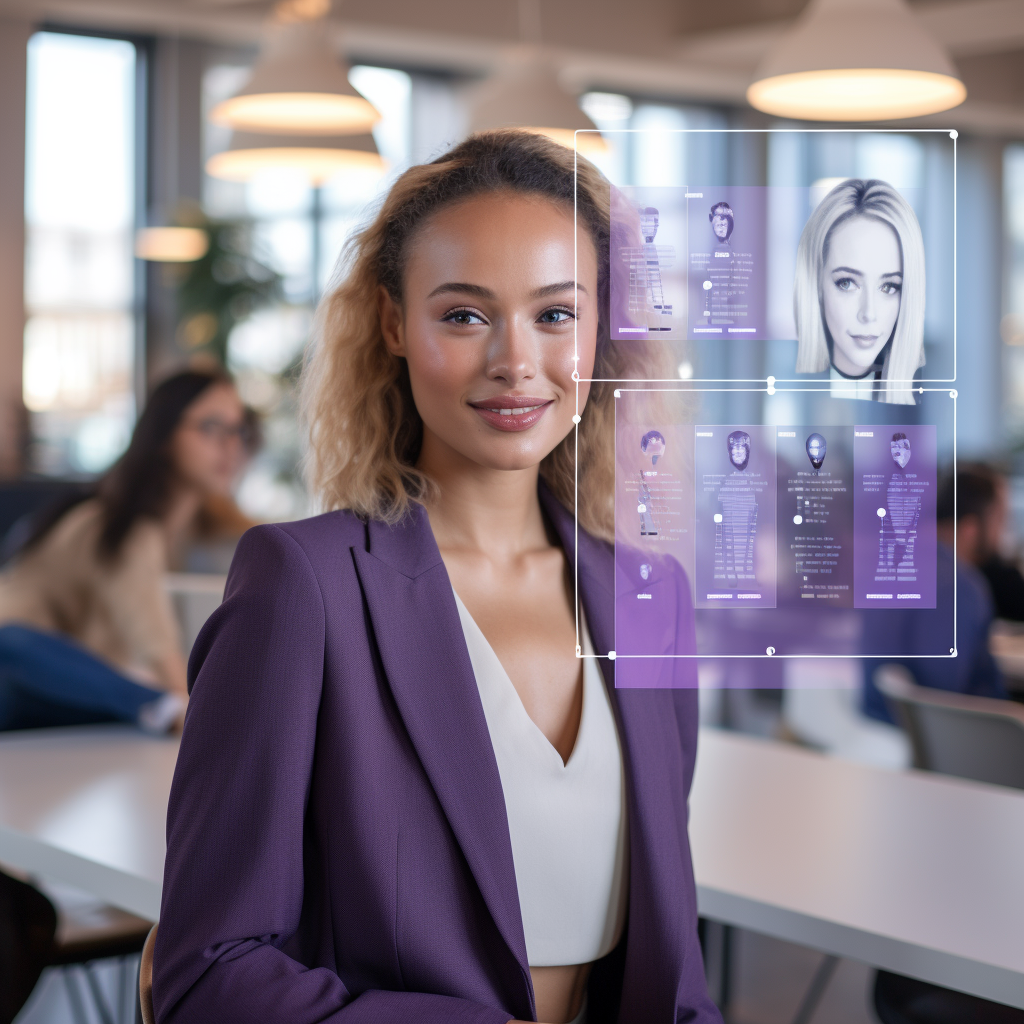
The Shortcomings of Traditional LLM Approaches
Traditional approaches towards building LLMs have their limitations when it comes to personalization. Sequence-to-sequence models, though effective in generating high-quality text, require immense computational power and are not inherently built to pull user-specific data. On the other hand, retrieval-based models, which search and retrieve the most appropriate response from a pre-defined dataset, offer limited scope for real-time personalization.
Additionally, when applied at scale, both these methods demand massive infrastructural investment, making it an expensive proposition for most enterprises.
What is Retrieval-Augmented Generation (RAG)?
Retrieval-Augmented Generation is a hybrid approach that combines the best of both retrieval-based and generative models. A paper by Facebook AI Research introduced this concept, highlighting its efficiency and effectiveness.
In RAG, the system initially uses a retrieval model to fetch relevant ‘passages’ or ‘snippets’ from a large corpus of data. These retrieved passages are then used as additional context for a sequence-to-sequence model that generates the final response. This results in highly accurate, context-rich, and personalized text generation.
How RAG Makes Personalization Cost-Effective
One of the biggest advantages of RAG is its economic viability, primarily due to the following reasons:
- Reduced Computational Load: By breaking down the process into retrieval and generation, RAG reduces the computational load required for each task. This makes it possible to run high-quality LLMs on less powerful hardware.
- Optimized Data Usage: RAG uses a large corpus of data more efficiently by retrieving only the relevant snippets, thus significantly cutting down the amount of data needed for training and inference.
- Scalability: Due to its modular architecture, RAG allows enterprises to scale their LLMs effortlessly, catering to increasing user demands without proportionally increasing costs.
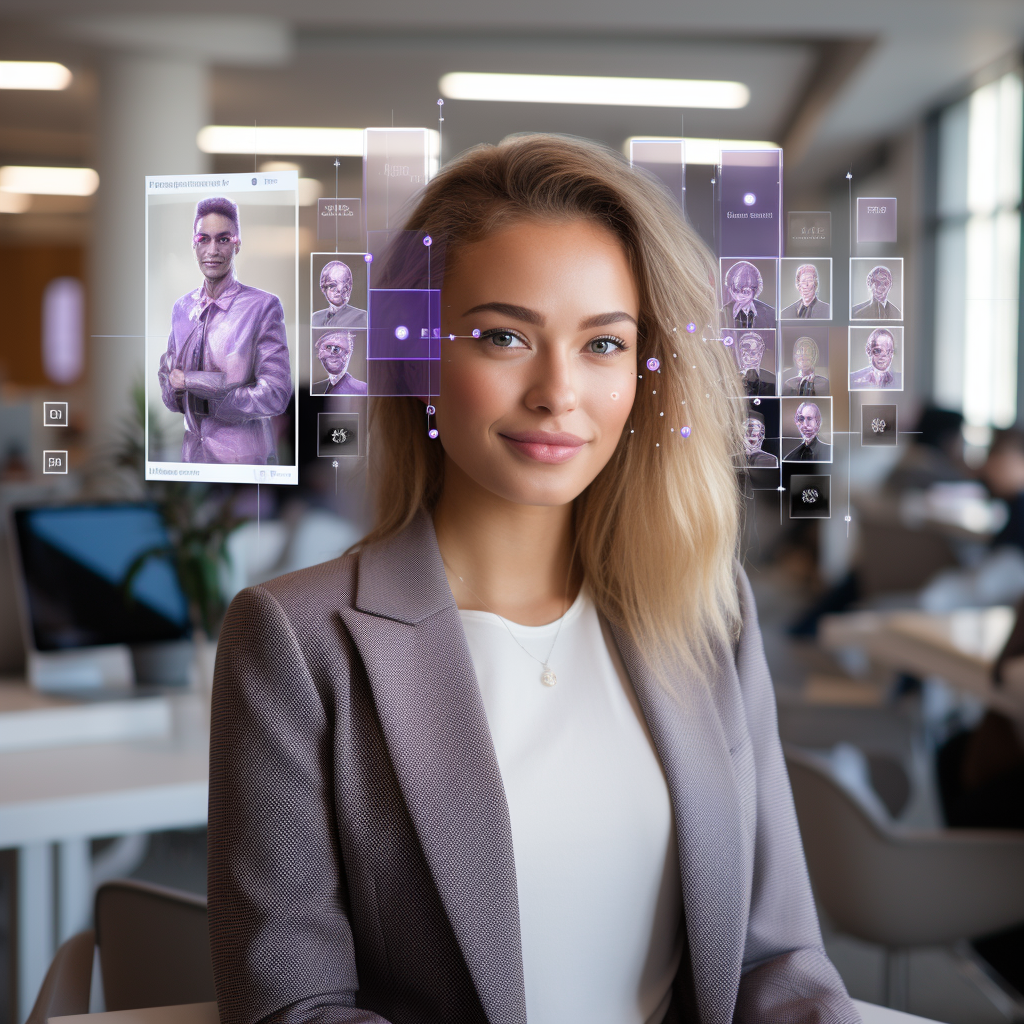
The Future of Personalization with RAG
Given the early successes and the growing research around RAG, it’s evident that the future of personalized LLM experiences lies in the broader adoption of this technology. Companies can look forward to providing hyper-personalized services, from customer support to content recommendation, without breaking the bank.
Here at Particlesy, we are continually fine-tuning our AI platform to leverage RAG for providing cost-effective, yet powerful, personalized experiences to our users.
In conclusion, Retrieval-Augmented Generation offers an exciting pathway for businesses looking to offer personalized services via LLMs without incurring astronomical costs. As computational techniques continue to evolve, RAG stands as a pioneering approach that combines efficiency with quality, paving the way for the next generation of personalized digital experiences.
For more insights into how our Particlesy platform uses RAG to deliver personalized experiences, contact us today.